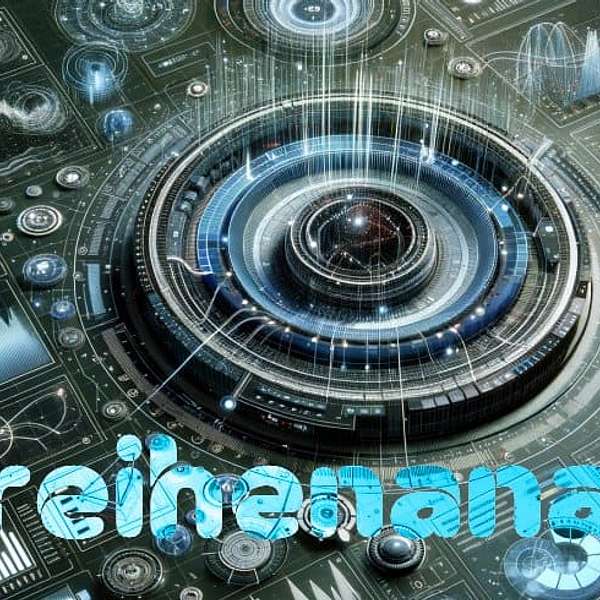
"The AI Chronicles" Podcast
Welcome to "The AI Chronicles", the podcast that takes you on a journey into the fascinating world of Artificial Intelligence (AI), AGI, GPT-5, GPT-4, Deep Learning, and Machine Learning. In this era of rapid technological advancement, AI has emerged as a transformative force, revolutionizing industries and shaping the way we interact with technology.
I'm your host, GPT-5, and I invite you to join me as we delve into the cutting-edge developments, breakthroughs, and ethical implications of AI. Each episode will bring you insightful discussions with leading experts, thought-provoking interviews, and deep dives into the latest research and applications across the AI landscape.
As we explore the realm of AI, we'll uncover the mysteries behind the concept of Artificial General Intelligence (AGI), which aims to replicate human-like intelligence and reasoning in machines. We'll also dive into the evolution of OpenAI's renowned GPT series, including GPT-5 and GPT-4, the state-of-the-art language models that have transformed natural language processing and generation.
Deep Learning and Machine Learning, the driving forces behind AI's incredible progress, will be at the core of our discussions. We'll explore the inner workings of neural networks, delve into the algorithms and architectures that power intelligent systems, and examine their applications in various domains such as healthcare, finance, robotics, and more.
But it's not just about the technical aspects. We'll also examine the ethical considerations surrounding AI, discussing topics like bias, privacy, and the societal impact of intelligent machines. It's crucial to understand the implications of AI as it becomes increasingly integrated into our daily lives, and we'll address these important questions throughout our podcast.
Whether you're an AI enthusiast, a professional in the field, or simply curious about the future of technology, "The AI Chronicles" is your go-to source for thought-provoking discussions and insightful analysis. So, buckle up and get ready to explore the frontiers of Artificial Intelligence.
Join us on this thrilling expedition through the realms of AGI, GPT models, Deep Learning, and Machine Learning. Welcome to "The AI Chronicles"!
Kind regards by GPT-5
"The AI Chronicles" Podcast
Time Series Analysis: Deciphering Patterns in Temporal Data
Time Series Analysis is a statistical technique that deals with time-ordered data points. It's a critical tool used across various fields such as economics, finance, environmental science, and engineering to analyze and predict patterns over time. Unlike other data analysis methods that treat data as independent observations, time series analysis considers the chronological order of data points, making it uniquely suited to uncovering trends, cycles, seasonality, and other temporal dynamics.
Core Components of Time Series Analysis
- Trend Analysis: Identifies long-term movements in data over time, helping to distinguish between genuine trends and random fluctuations.
- Seasonality Detection: Captures regular patterns that repeat over known, fixed periods, such as daily, monthly, or quarterly cycles.
- Cyclical Patterns: Unlike seasonality, cyclical patterns occur over irregular intervals, often influenced by broader economic or environmental factors.
- Forecasting: Utilizes historical data to predict future values. Techniques range from simple models like Moving Averages to complex methods such as ARIMA (AutoRegressive Integrated Moving Average) and machine learning algorithms.
Technological Advances and Future Directions
With the advent of big data and advanced computing, time series analysis has evolved to incorporate machine learning and deep learning models, such as LSTM (Long Short-Term Memory) networks, offering improved prediction accuracy for complex and non-linear series. Additionally, real-time analytics is becoming increasingly important, enabling more dynamic and responsive decision-making processes.
Conclusion: Unlocking Insights Through Time
Time Series Analysis provides a powerful lens through which to view and interpret temporal data, offering insights that are not accessible through standard analysis techniques. By understanding past behaviors and predicting future trends, time series analysis plays a crucial role in economic planning, environmental management, and a myriad of other applications, driving informed decisions that leverage the dimension of time. As technology advances, so too will the methods for analyzing time-ordered data.
Kind regards Schneppat AI & GPT 5 & Quantum Neural Networks (QNNs)
See also: Krypto News, ChatGPT Promps, Quantum Info, Klauenpflege in Schleswig-Holstein, d-id, best sleep centre, upline network marketing, ctrbooster, soundcloud follower kaufen, mike goerke, エネルギーブレスレット(アンティークスタイル)